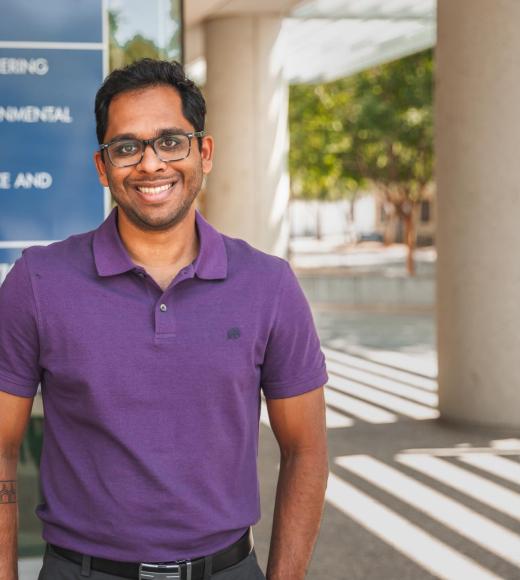
Position Title
University of California, Davis
Prof. Ambarish Kulkarni
Abstract
Theory-guided characterization of atomically dispersed transition metal catalysts
The democratization of machine learning (ML) and the advent of open-access data repositories have revolutionized materials science in the preceding decade. These advances have enabled high-throughput in-silico screening of large materials libraries, but the measurable impact on laboratory-scale development of functional materials and novel processes is still limited. Why? In part because of the disconnect between theoretical “models” and real-world experimental “samples.” For example, the promising active site environment of a catalyst (often predicted by theory) is often far different from what is synthesized in practice. A high-priority need is thus to develop integrated materials development workflows that bridge (1) computational materials design, (2) laboratory-scale materials synthesis, (3) unbiased experimental characterization, and (4) rapid performance evaluation. Recognizing these bottlenecks, this talk will demonstrate unique insights provided by theory in collaboration with experimentalists. An example discussed in detail is the development of a theory-based approach to fitting Extended X-Ray Absorption Fine Structure (EXAFS) data, providing unprecedented structural detail and confidence in the local binding environment for “single-atom” catalysts like Pt/MgO. More broadly, this talk will present a cohesive vision for the expanding role of theory and data science (used judiciously in conjunction with experimentation) to understand the structure, evolution, and reactivity of atomically dispersed catalysts.
Biography
Ambar Kulkarni is an Assistant Professor in the Department of Chemical Engineering at UC Davis. His research is focused on combining advances in multiscale molecular modeling and machine learning with multifaceted experimental collaborations to addresses big challenges in catalysis, separations, and sensing. The Kulkarni group is supported through grants from the National Science Foundation (NSF), the Department of Energy (DOE), the Center for Data Science and Artificial Intelligence Research (CEDAR UC Davis), and industry partnerships.
Kulkarni’s interdisciplinary research was recognized with the 2021 UC Davis College of Engineering Outstanding Junior Faculty Award for Excellence in Research. He received a 2021 NSF CAREER award for his work in designing MOFs for electrochemical applications. As the Inaugural UC Davis Graduate Mentor Fellow (2020-2022), Kulkarni has developed a series of mentorship workshops for UC Davis faculty aimed at improving the graduate student mentorship experience.